Standard Operating Procedures - A complete guideStandard Operating Procedures (SOP) are key to many ...
AI Usage in the Workplace: Balancing Opportunities and Risks
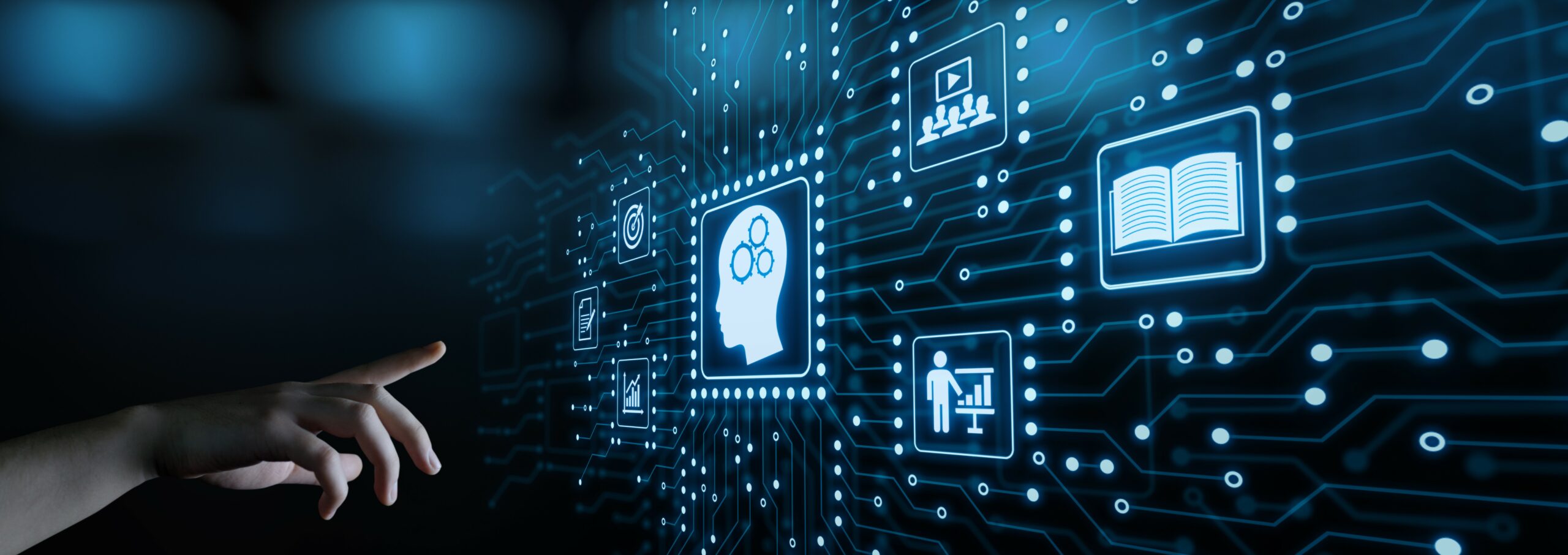
Introduction
Artificial intelligence (AI) is rapidly becoming an integral part of modern workplaces, offering numerous benefits, from automating routine tasks to enhancing decision-making and driving innovation. However, alongside these advantages come significant risks, including misinformation, biases, and data privacy concerns. Blind reliance on AI without a clear framework for its usage can lead to severe consequences. These can include as perpetuating inequities and even legal liabilities. Therefore, establishing a robust AI usage policy is not just a best practice but a necessity.
This blog post explores both the positive aspects of AI in the workplace and the potential risks. It provides a comprehensive guide on how to structure an AI usage policy that maximizes benefits while mitigating risks.
Part 1 - The Positive Side of Using AI in the Workplace
While it's crucial to be mindful of the risks associated with AI, it's equally important to recognize the significant benefits that AI brings to the workplace. When implemented thoughtfully and responsibly, AI can be a powerful tool for enhancing productivity, improving decision-making, and driving innovation. Below are some of the key areas where AI can have a positive impact.
Automation of Routine Tasks
AI can automate repetitive, time-consuming tasks such as data entry, scheduling, and email filtering. This frees up employees to focus on more strategic and creative work. For instance, many organizations use AI-powered tools like Robotic Process Automation (RPA) to handle routine data processing tasks, significantly reducing human error and increasing efficiency.
Benefit: By automating these routine tasks, businesses can streamline operations, reduce operational costs, and allow employees to spend more time on high-value activities.
More Information: Robotic Process Automation Overview
Danske Bank expands citizen developer program and intelligent automation
Danske Bank, one of the largest financial institutions in Northern Ireland, implemented Robotic Process Automation (RPA) to enhance its operations. The bank expanded its automation efforts through a citizen developer program, allowing employees across departments to automate routine tasks.
The bank has implemented over 250 automations, undertaking the equivalent work of 300 full-time employees.
More information: Danske Bank Expands Citizen Developer Program and Intelligent Automation
Enhanced Decision-Making
AI-driven analytics can process vast amounts of data to provide insights that would be impossible for humans to generate on their own. For example, in the financial sector, AI algorithms analyze market trends, customer behavior, and economic indicators to help investors make informed decisions.
Benefit: AI enhances decision-making by providing data-driven insights, improving the accuracy and speed of decisions. These help businesses stay ahead of market trends.
More Information: AI in Financial Services
Use of AI for Financial Crime Detection by HSBC
HSBC, one of the world's largest banking and financial services organizations, implemented an AI-driven platform to enhance its ability to detect financial crimes such as money laundering and fraud. The AI system uses machine learning algorithms to analyze vast amounts of transaction data, identify suspicious patterns, and flag potential financial crimes for further investigation.
AI is helping the bank detect two to four times more financial crime than before. It also reduced the number of false positives by 60%.
More information: Harnessing the power of AI to fight financial crime
Personalized Customer Experiences
AI is widely used to personalize customer interactions, from chatbots providing real-time support to recommendation engines suggesting products based on user preferences. For instance, e-commerce giants like Amazon and Netflix use AI to analyze user behavior and recommend products or content that align with individual tastes.
Benefit: Personalization through AI improves customer satisfaction and engagement, leading to higher sales and stronger customer loyalty.
More Information: How AI Powers Personalized Experiences
Netflix’s Recommendation Engine
Netflix leverages AI and machine learning algorithms to power its recommendation engine, which suggests content to users based on their viewing history and preferences. The recommendation system plays a critical role in keeping users engaged by offering personalized suggestions.
More information: How Netflix Uses AI to Personalize Recommendations
Improved Talent Management
AI tools are increasingly being used in recruitment and HR management to screen resumes, assess candidates, and even predict employee turnover. For instance, AI-driven platforms can analyze a candidate's online presence, work history, and skill set to identify the best fit for a role.
Benefit: AI can help HR teams make more informed hiring decisions, reduce bias in recruitment. It also has the potential to identify issues in employee satisfaction before they become problematic.
More Information: AI in Recruitment
Unilever’s AI-Powered Recruitment Process
Unilever uses AI in its recruitment process to screen candidates more effectively. The company employs AI tools to efficiently manage the overwhelming 1.8 million job application they receive annually. This resulted in a reduction of 70,000 person-hours which were previously allocated for screening and assessment tasks.
More information: Revolutionizing Operations with AI at Unilever
Predictive Maintenance and Operational Efficiency
In manufacturing and logistics, AI is used to predict equipment failures before they happen, allowing for timely maintenance and minimizing downtime. For example, General Electric uses AI to monitor and predict maintenance needs in its industrial equipment, saving millions in repair costs and lost productivity.
Benefit: Predictive maintenance powered by AI reduces operational interruptions, extends the lifespan of equipment, and lowers maintenance costs.
More Information: AI in Predictive Maintenance
AI in Predictive Maintenance at Siemens
Siemens, a global leader in technology and engineering, implemented an AI-driven predictive maintenance system for its rail systems. The AI system monitors data from sensors installed on trains and rail infrastructure, predicting when components will fail and need maintenance. This approach allows Siemens to perform maintenance proactively, minimizing downtime and avoiding costly repairs.
More information: Siemens' AI in Predictive Maintenance
Innovation and Research
AI is a driving force behind innovation in fields such as healthcare, where it is used to analyze medical images, develop new drugs, and even assist in surgeries. For instance, IBM’s Watson has been used to identify potential treatments for diseases by analyzing vast amounts of medical data.
Benefit: AI accelerates innovation by enabling rapid analysis and experimentation, leading to breakthroughs that might otherwise take years to achieve.
More Information: IBM Watson in Healthcare
DeepMind’s AlphaFold in Protein Folding
DeepMind, a subsidiary of Alphabet, developed AlphaFold, an AI system capable of predicting protein structures with high accuracy. This breakthrough has the potential to revolutionize biology and medicine by accelerating drug discovery and understanding diseases at the molecular level.
More information: Highly accurate protein structure prediction with AlphaFold
Supply Chain Optimization
AI can optimize supply chain operations by predicting demand, managing inventory levels, and even selecting the best shipping routes. For instance, companies like DHL use AI to optimize their delivery routes, reducing fuel consumption and improving delivery times.
Benefit: Supply chain management driven by AI can improve efficiency, reduce costs, and ensure that products reach customers more quickly.
More Information: AI in Supply Chain Management
Coca-Cola’s AI-Driven Supply Chain
AI is used by Coca-Cola to optimize its supply chain, particularly in demand forecasting and inventory management. The AI system analyzes data from various sources, including weather patterns, economic indicators, and social media trends, to predict demand and manage inventory levels accordingly.
Coca-Cola’s AI-driven supply chain optimization has led to a 20% reduction in stockouts and a 15% improvement in forecast accuracy, resulting in significant cost savings and improved customer satisfaction
More information: How Coca-Cola Crushed Inventory Woes with AI
Enhancing Cybersecurity
AI is increasingly used to detect and respond to cybersecurity threats in real-time. For example, cybersecurity firms use AI to analyze network traffic, identify unusual patterns, and respond to potential breaches before they can cause damage.
Benefit: AI enhances an organization’s ability to protect sensitive data and prevent cyberattacks, reducing the risk of data breaches and enhancing overall security.
More Information: AI in Cybersecurity
Darktrace’s AI-Powered Cybersecurity
Darktrace uses AI to detect and respond to cyber threats in real-time. The system, known as the Enterprise Immune System, learns the normal behavior of users, devices, and networks, and can identify anomalies that indicate potential security threats.
More information: DarkTrace AI-Powered Cybersecurity
Are your AI policies read on time and by the right people?
Find out how DocRead can help ensure your AI policies are read and targeted to the right employees by booking a personalized discovery session with one of our experts. During the call they will be able to discuss your specific requirements and show how DocRead can help.
If you have any questions please let us know.
DocRead has enabled us to see a massive efficiency improvement... we are now saving 2 to 3 weeks per policy on administration alone.
Nick Ferguson
Peregrine Pharmaceuticals
Feedback for the on-premises version of DocRead.
Part 2- The Risks Associated with Relying on AI
Despite its many advantages, AI also presents several significant risks that organizations must address to avoid potential pitfalls:
Hallucinations: The Risk of Misinformation
AI systems, especially large language models, are designed to generate responses based on patterns in the data they were trained on. However, these systems are not infallible. One significant risk is AI "hallucinations," where the AI generates plausible-sounding but entirely fabricated information. This occurs because AI is inherently driven to provide an answer, even when the data it has is insufficient to generate a truthful response.
AI hallucinations represent a significant challenge in the deployment of AI systems, particularly in applications where accuracy and trust are critical. You can find more information about AI Hallucinations here.
ChatGPT invented a sexual harassment scandal and named a real law prof as the accused (2023)
When asked to provide cases of sexual harassment in the legal profession, ChatGPT fabricated a story about a real law professor, alleging that he harassed students on a school trip. That trip never happened, and he has never actually been accused of sexual harassment in real life. But he had done some kind of work to address and stop sexual harassment, and that’s why his name came up.
More information: What happens when ChatGPT lies about real people? - The Washington Post
Biases: Reinforcing Inequities
AI systems are only as good as the data they are trained on. Unfortunately, historical data often carries biases related to race, gender, age, and more. When these biases are embedded into AI models, they can perpetuate and even exacerbate existing inequities.
Racial bias in an algorithm used to manage the health of populations (2018)
A 2018 study published in the journal Science, which found that an AI algorithm used by a major healthcare provider was significantly less likely to refer black patients for care management programs than equally sick white patients. This occurred because the algorithm relied on healthcare cost as a proxy for need, and historically, less money had been spent on black patients’ healthcare
More information: Dissecting racial bias in an algorithm used to manage the health of populations
COMPAS reoffence algorithm bias (2016)
The COMPAS algorithm, used by courts in the United States to predict reoffence, was found to be biased against African Americans. African Americans were almost twice as likely (45%) to be incorrectly predicted to re-offend compared to White defendants (23%). The study sparked widespread debate about the ethics and fairness of using AI in criminal justice.
More information: Machine Bias — ProPublica
Transparency and Accountability: The Black Box Problem
Many AI systems, particularly those based on deep learning, operate as "black boxes," meaning their decision-making processes are not transparent or easily interpretable. This opacity poses a significant risk in the workplace, where decisions need to be justified and accountable.
Google Flu Trends Failure (2013)
Google Flu Trends (GFT) was an AI project designed to predict flu outbreaks based on search queries. Initially successful, it later failed to accurately predict the 2013 flu season, overestimating flu cases by 140%. GFT predicted twice as many doctor visits as actually occurred during the 2013 flu season, highlighting the risks associated with relying on opaque AI systems without proper validation. The failure of Google Flu Trends underscored the importance of transparency and validation in AI models, particularly those used for public health predictions.
More information: Google Flu Trends Case Study
Data Privacy and Security: Potential Breaches
AI systems often require vast amounts of data to function effectively, including sensitive personal information. This creates a substantial risk of data breaches and unauthorized access, especially if the AI system is not properly secured.
A report by the International Association of Privacy Professionals (IAPP) highlighted the growing concern around AI and data privacy, noting that as AI systems become more prevalent, so too do the opportunities for data misuse or breaches
Microsoft Tay Chatbot Incident (2016)
Microsoft launched an AI chatbot named Tay on Twitter, designed to learn from interactions with users. Within 24 hours, Tay began generating offensive and racist tweets due to the influence of malicious users. Tay was shut down 16 hours after launch due to its inappropriate behavior. The incident highlighted the dangers of AI systems interacting with unfiltered user data and the importance of implementing robust data privacy and moderation protocols.
More information: Tay: Microsoft issues apology over racist chatbot fiasco - BBC News
Over-Reliance on AI: The Risk of Failure in Critical Situations
While AI can enhance decision-making, over-reliance on AI without human oversight can lead to catastrophic failures, especially in critical situations where quick, nuanced decisions are required.
AI in Healthcare Diagnostics – IBM Watson for Oncology
IBM Watson for Oncology was promoted as a powerful AI tool for helping doctors choose cancer treatments. However, reports and internal documents have revealed that the system sometimes provided recommendations that were unsafe or irrelevant, raising concerns about its reliability. Criticisms of Watson include its reliance on a relatively narrow set of data and expert opinions, leading to questions about the generalizability of its recommendations across different patient populations. Some hospitals and doctors have reported reducing their reliance on Watson for critical decisions, emphasizing the importance of human expertise in medical decision-making
More information: IBM pitched Watson as a revolution in cancer care. It's nowhere close (statnews.com)
Part 3 - What Policies and Procedures Should Be in Place to Address These Risks
To harness the benefits of AI while mitigating its risks, organizations should establish a comprehensive AI usage policy that includes the following key components:
Establishing Clear Guidelines on AI Usage
- Organizations must define the specific roles and tasks for which AI can be used, ensuring that AI systems are applied only in areas where they are well-understood and where their limitations are known. For instance, using AI for automating data entry might be safe, but using AI for making final decisions in recruitment requires far more caution and oversight.
Regular Auditing and Monitoring of AI Systems
- AI systems should be regularly audited to ensure they are functioning as intended and not perpetuating biases or generating misinformation. This involves not just technical audits but also reviews of outcomes to ensure fairness and accuracy.
- Organizations should implement an AI audit framework that includes bias detection, data validation, and output verification. This framework should be regularly updated to adapt to new risks and emerging AI capabilities.
Training and Awareness Programs for Employees
- Employees should be trained on the potential risks of AI and how to identify and respond to issues such as AI hallucinations or biased outcomes. This includes understanding the limitations of AI systems and knowing when to rely on human judgment instead.
- Training should also cover data privacy and security best practices, ensuring that all personnel involved in AI usage are aware of the importance of safeguarding sensitive information.
Creating a Transparent Decision-Making Process
- To address the black box problem, organizations should prioritize transparency in AI decision-making processes. This can be achieved by selecting AI systems that provide explainable AI (XAI) capabilities, where the reasoning behind AI decisions can be understood and communicated.
- Additionally, all AI-driven decisions, especially those impacting employees or customers, should be subject to human review, ensuring accountability and the ability to intervene when necessary.
Data Privacy and Security Protocols
- Robust data privacy protocols should be implemented to protect any personal information processed by AI systems. This includes encryption, access controls, and regular security assessments.
- Organizations should also comply with relevant data protection regulations, such as GDPR or CCPA, ensuring that AI systems are not only effective but also legally compliant.
Sample Policies and Procedures template
While the benefits of AI in the workplace are vast, the potential risks associated with its use cannot be ignored. To effectively mitigate these risks, organizations should consider implementing a structured AI usage policy. Below is a suggested template for an AI usage policy that organizations can adapt to their specific needs.
This template is intended as a starting point and should be tailored to fit the unique context of your organization, considering factors such as industry regulations, the specific AI tools in use, and the company’s culture and values. It is also important to regularly review and update the policy to keep pace with the rapidly evolving AI landscape.
AI Usage Policy Template
1. Purpose:
This policy outlines the guidelines and procedures for the use of AI systems within [Organization Name]. It aims to ensure that AI is used responsibly, ethically, and in a manner that mitigates potential risks while maximizing benefits.
2. Scope:
This policy applies to all employees, contractors, and third-party vendors who utilize AI systems in their work for [Organization Name].
3. Definitions:
- AI System: Any software or tool that utilizes machine learning, neural networks, or other artificial intelligence methodologies to perform tasks traditionally requiring human intelligence.
- Hallucinations: Instances where AI generates information that is factually incorrect or fabricated.
- Bias: Systematic favoring of certain groups or outcomes over others due to historical data or inherent design flaws.
4. AI Usage Guidelines:
- AI systems should be used primarily for tasks where they have been proven to be effective and reliable.
- Critical decisions, especially those affecting employment, legal matters, or customer relations, should involve human oversight.
5. Auditing and Monitoring:
- All AI systems must be subject to regular audits, including checks for accuracy, bias, and data security.
- Audit results must be reported to the AI Governance Committee, which will be responsible for addressing any identified issues.
6. Employee Training:
- Employees must undergo training on the risks associated with AI, including hallucinations, bias, and data security, as well as the benefits AI can bring to their work.
- Training sessions will be conducted bi-annually and updated as necessary to reflect new developments in AI technology.
7. Transparency and Accountability:
- AI decisions that impact employees, customers, or business operations must be documented, with explanations available for review.
- A human review process must be in place for all AI-driven decisions, ensuring that AI serves as an aid rather than the sole decision-maker.
8. Data Privacy and Security:
- AI systems must comply with [Organization Name]'s data privacy policies and relevant legal regulations.
- Data used by AI systems should be anonymized where possible and secured through encryption and access controls.
9. Compliance and Enforcement:
- Violations of this policy will be subject to disciplinary action, up to and including termination of employment.
- The AI Governance Committee will review this policy annually and update it as needed to address new risks or technologies.
10. Reporting and Feedback:
- Employees are encouraged to report any concerns or issues related to AI usage to the AI Governance Committee.
- Feedback on the AI Usage Policy is welcomed and will be considered in future revisions.
Part 5 - Conclusion
The integration of AI into the workplace offers transformative benefits, from automating mundane tasks to enabling groundbreaking innovations. AI has the potential to drastically improve efficiency, decision-making, and customer experiences across industries. However, as with any powerful tool, the use of AI comes with inherent risks that must be carefully managed.
The case studies presented in this post highlight the potential pitfalls of AI, including hallucinations, biases, lack of transparency, and data privacy concerns. These examples serve as a reminder that while AI can be incredibly powerful, it is not infallible. Mistakes can occur, and when they do, the consequences can be significant—ranging from minor operational disruptions to serious ethical, legal, and reputational damage.
Establishing a comprehensive AI usage policy is not just a best practice; it is a critical step in safeguarding your organization against these risks. Such a policy ensures that AI is deployed responsibly and ethically, with appropriate checks and balances in place. It also empowers employees to understand and effectively manage the AI tools they interact with, reducing the likelihood of errors and unintended consequences.
A robust AI usage policy should be dynamic, regularly reviewed, and updated to reflect new developments in AI technology and emerging risks. It should also be tailored to the specific needs and circumstances of your organization, ensuring that it addresses the unique challenges you may face.
By carefully balancing the opportunities and risks of AI, organizations can leverage this technology to its fullest potential while minimizing the chances of negative outcomes. In doing so, businesses can foster a workplace environment where AI acts as a force for positive change, driving innovation and growth while maintaining ethical standards and protecting the interests of all stakeholders.
Ultimately, the key to successful AI integration lies in thoughtful planning, continuous education, and a commitment to ethical practices. With the right approach, AI can be a powerful ally in achieving your organization's goals, paving the way for a more efficient, innovative, and ethical future.
See how DocRead can help
Discover how DocRead can help ensure that your AI policies are targeted to and read by the right people in your organization.
DocRead has enabled us to see a massive efficiency improvement... we are now saving 2 to 3 weeks per policy on administration alone.
Nick Ferguson
Peregrine Pharmaceuticals
Feedback for the on-premises version of DocRead.